AI could help solve the privacy problems it has created
Artificial intelligence insatiable data needs has encouraged the mass collection of personal data, placing privacy at risk. But AI can help solve the very problem it creates.
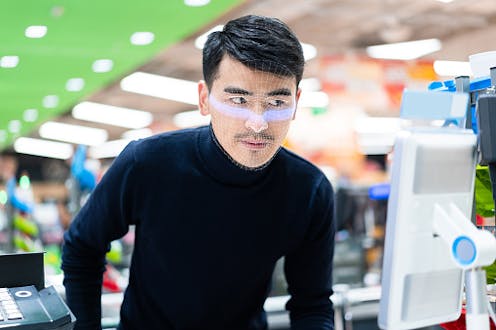
The stunning successes of artificial intelligence would not have happened without the availability of massive amounts of data, whether its smart speakers in the home or personalized book recommendations. And the spread of AI into new areas of the economy, such as AI-driven marketing and self driving vehicles, has been driving the collection of ever more data. These large databases are amassing a wide variety of information, some of it sensitive and personally identifiable. All that data in one place makes such databases tempting targets, ratcheting up the risk of privacy breaches.
The general public is largely wary of AI’s data-hungry ways. According to a survey by Brookings, 49% of people think AI will reduce privacy. Only 12% think it will have no effect, and a mere 5% think it may make it better.
As cybersecurity and privacy researchers, we believe that the relationship between AI and data privacy is more nuanced. The spread of AI raises a number of privacy concerns, most of which people may not even be aware. But in a twist, AI can also help mitigate many of these privacy problems.
Revealing models
Privacy risks from AI stem not just from the mass collection of personal data, but from the deep neural network models that power most of today’s artificial intelligence. Data isn’t vulnerable just from database breaches, but from “leaks” in the models that reveal the data on which they were trained.
Deep neural networks – which are a collection of algorithms designed to spot patterns in data – consist of many layers. In those layers are a large number of nodes called neurons, and neurons from adjacent layers are interconnected. Each node, as well as the links between them, encode certain bits of information. These bits of information are created when a special process scans large amounts of data to train the model.
For example, a facial recognition algorithm may be trained on a series of selfies so it can more accurately predict a person’s gender. Such models are very accurate, but they also may store too much information – actually remembering certain faces from the training data. In fact, that’s exactly what researchers at Cornell University discovered. Attackers could identify people in training data by probing the deep neural networks that classified the gender of facial images.
They also found that even if the original neural network model is not available to attackers, attackers may still be able to tell whether a person is in the training data. They do this by using a set of models that are trained on data similar, but not identical, to the training data. So if a man with a beard was present in the original training data, then a model trained on photos of different bearded men may be able to reveal his identity.
AI to the rescue?
On the other hand, AI can be used to mitigate many privacy problems. According to Verizon’s 2019 Data Breach Investigations Report, about 52% of data breaches involve hacking. Most existing techniques to detect cyberattacks rely on patterns. By studying previous attacks, and identifying how the attacker’s behavior deviates from the norm, these techniques can flag suspicious activity. It’s the sort of thing at which AI excels: studying existing information to recognize similar patterns in new data.
Still, AI is no panacea. Attackers can often modify their behavior to evade detection. Take the following two examples. For one, suppose anti-malware software uses AI techniques to detect a certain malicious program by scanning for a certain sequence of software code. In that case, an attacker can simply shuffle the order the code. In another example, the anti-malware software might first run the suspicious program in a safe environment, called a sandbox, where it can look for any malicious behavior. Here, an attacker can instruct the malware to detect if it’s being run in a sandbox. If it is, it can behave normally until it’s released from the sandbox – like a possum playing dead until the threat has passed.
Making AI more privacy friendly
A recent branch of AI research called adversarial learning seeks to improve AI technologies so they’re less susceptible to such evasion attacks. For example, we have done some initial research on how to make it harder for malware, which could be used to violate a person’s privacy, to evade detection. One method we came up with was to add uncertainty to the AI models so the attackers cannot accurately predict what the model will do. Will it scan for a certain data sequence? Or will it run the sandbox? Ideally, a malicious piece of software won’t know and will unwittingly expose its motives.
Another way we can use AI to improve privacy is by probing the vulnerabilities of deep neural networks. No algorithm is perfect, and these models are vulnerable because they are often very sensitive to small changes in the data they are reading. For example, researchers have shown that a Post-it note added to a stop sign can trick an AI model into thinking it is seeing a speed limit sign instead. Subtle alterations like that take advantage of the way models are trained to reduce error. Those error-reduction techniques open a vulnerability that allows attackers to find the smallest changes that will fool the model.
These vulnerabilities can be used to improve privacy by adding noise to personal data. For example, researchers from Max Planck Institute for Informatics in Germany have designed clever ways to alter Flickr images to foil facial recognition software. The alterations are incredibly subtle, so much so that they’re undetectable by the human eye.
The third way that AI can help mitigate privacy issues is by preserving data privacy when the models are being built. One promising development is called federated learning, which Google uses in its Gboard smart keyboard to predict which word to type next. Federated learning builds a final deep neural network from data stored on many different devices, such as cellphones, rather than one central data repository. The key benefit of federated learning is that the original data never leaves the local devices. Thus privacy is protected to some degree. It’s not a perfect solution, though, because while the local devices complete some of the computations, they do not finish them. The intermediate results could reveal some data about the device and its user.
Federated learning offers a glimpse of a future where AI is more respectful of privacy. We are hopeful that continued research into AI will find more ways it can be part of the solution rather than a source of problems.
[You’re smart and curious about the world. So are The Conversation’s authors and editors. You can read us daily by subscribing to our newsletter.]
Zhiyuan Chen receives funding from National Science Foundation, Office of Naval Research, National Geospatial-Intelligence Agency, NIST, US Department of Education, IBM, and TEDCO.
Aryya Gangopadhyay receives funding from the National Science Foundation, Department of Education, and IBM.
Read These Next
How artificial intelligence controls your health insurance coverage
Health insurance companies use AI to decide which health care treatment to cover. State laws and federal…
At Antarctica’s midwinter, a look back at the frozen continent’s long history of dark behavior
Antarctica is a harsh continent, which in both history and fiction can trigger disturbing behavior and…
Trump administration’s conflicting messages on Chinese student visas reflect complex US-China relati
The Trump administration’s threat to revoke visas of some Chinese students isn’t the first time…