AI tool searches thousands of scientific papers to guide researchers to coronavirus insights
The scientific community is churning out vast quantities of research about the coronavirus pandemic – far too much for researchers to absorb. An AI system aims to do the heavy lifting for them.
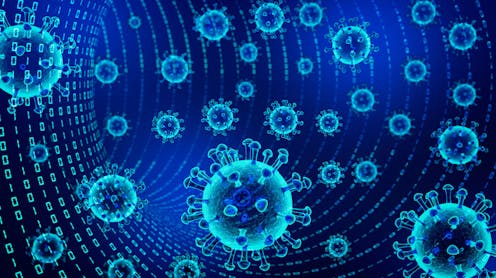
The Research Brief is a short take about interesting academic work.
The big idea
The scientific community worldwide has mobilized with unprecedented speed to tackle the COVID-19 pandemic, and the emerging research output is staggering. Every day, hundreds of scientific papers about COVID-19 come out, in both traditional journals and non-peer-reviewed preprints. There’s already far more than any human could possibly keep up with, and more research is constantly emerging.
And it’s not just new research. We estimate that there are as many as 500,000 papers relevant to COVID-19 that were published before the outbreak, including papers related to the outbreaks of SARS in 2002 and MERS in 2012. Any one of these might contain the key information that leads to effective treatment or a vaccine for COVID-19.
Traditional methods of searching through the research literature just don’t cut it anymore. This is why we and our colleagues at Lawrence Berkeley National Lab are using the latest artificial intelligence techniques to build COVIDScholar, a search engine dedicated to COVID-19. COVIDScholar includes tools that pick up subtle clues like similar drugs or research methodologies to recommend relevant research to scientists. AI can’t replace scientists, but it can help them gain new insights from more papers than they could read in a lifetime.
Why it matters
When it comes to finding effective treatments for COVID-19, time is of the essence. Scientists spend 23% of their time searching for and reading papers. Every second our search tools can save them is more time to spend making discoveries in the lab and analyzing data.
AI can do more than just save scientists time. Our group’s previous work showed that AI can capture latent scientific knowledge from text, making connections that humans missed. There, we showed that AI was able to suggest new, cutting-edge functional materials years before their discovery by humans. The information was there all along, but it took combining information from hundreds of thousands of papers to find it.
We are now applying the same techniques to COVID-19, to find existing drugs that could be repurposed, genetic links that might help develop a vaccine or effective treatment regimens. We’re also starting to build in new innovations, like using molecular structures to help find which drugs are similar to each other, including those that are similar in unexpected ways.
How we do this work
The most important part of our work is the data. We’ve built web scrapers that collect new papers as they’re published from a wide variety of sources, making them available on our website within 15 minutes of their appearance online. We also clean the data, fixing mistakes in formatting and comparing the same paper from multiple sources to find the best version. Our machine learning algorithms then go to work on the paper, tagging it with subject categories and marking work important to COVID-19.
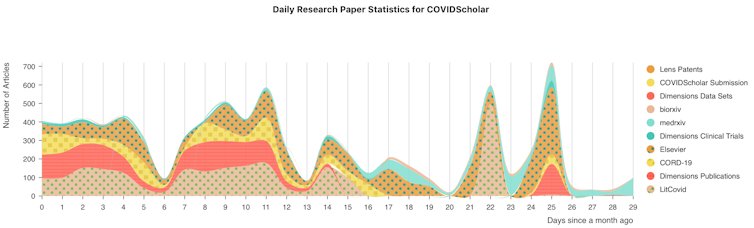
We’re also continuously seeking out experts in new areas. Their input and annotation of data is what allows us to train new AI models.
What’s next
So far, we have assembled a collection of over 60,000 papers on COVID-19, and we’re expanding the collection daily. We’ve also built search tools that group research into categories, suggest related research and allow users to find papers that connect different concepts, such as papers that connect a specific drug to the diseases it’s been used to treat in the past. We’re now building AI algorithms that allow researchers to plug search results into quantitative models for studying topics like protein interactions. We’re also starting to dig through the past literature to find hidden gems.
We hope that very soon, researchers using COVIDScholar will start to identify relationships that they might never have imagined, bringing us closer to treatments and a remedy for COVID-19.
[You’re too busy to read everything. We get it. That’s why we’ve got a weekly newsletter. Sign up for good Sunday reading. ]
Amalie Trewartha receives funding from the Department of Energy and has previously received funding from the Toyota Research Institute.
John Dagdelen works for Lawrence Berkeley National Laboratory. He receives funding from Berkeley Lab and previously from the Toyota Research Institute.
Read These Next
Myanmar military’s ‘ceasefire’ follows a pattern of ruling generals exploiting disasters to shore up
Thousands were killed in 7.7 magnitude earthquake. Yet military generals were slow to agree to pause…
How a lone judge can block a Trump order nationwide – and why, from DACA to DOGE, this judicial chec
Do court-imposed nationwide injunctions, which stop policies for everyone across the country, give too…
Trump’s ‘Liberation Day’ tariffs are the highest in decades − an economist explains how that could h
Foreign investment thrives on stability. Trump’s tariffs − and the current climate of uncertainty…