How governments handle data matters for inclusion
Governments can exclude certain groups of people in policies and services not only by the type of data they collect but also how they collect, store, analyze and use the data.
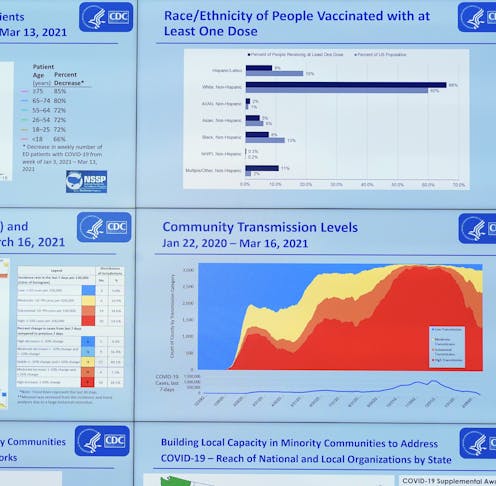
Governments increasingly rely on large amounts of data to provide services ranging from mobility and air quality to child welfare and policing programs. While governments have always relied on data, their increasing use of algorithms and artificial intelligence has fundamentally changed the way they use data for public services.
These technologies have the potential to improve the effectiveness and efficiency of public services. But if data is not handled thoughtfully, it can lead to inequitable outcomes for different communities because data gathered by governments can mirror existing inequalities. To minimize this effect, governments can make inclusion an element of their data practices.
To better understand how data practices affect inclusion, we – scholars of public affairs, policy and administration – break down government data practices into four activities: data collection, storage, analysis and use.
Collection
Governments collect data about all manner of subjects via surveys, registrations, social media and in real time via mobile devices such as sensors, cellphones and body cameras. These datasets provide opportunities to shape social inclusion and equity. For example, open data can be used as a spotlight to expose health disparities or inequalities in commuting.
At the same time, we found that poor-quality data can worsen inequalities. Data that is incomplete, outdated or inaccurate can result in the underrepresentation of vulnerable groups because they may not have access to the technology used to collect the data. Also, government data collection might lead to oversurveillance of vulnerable communities. Consequently, some people may choose to avoid contributing data to government institutions.
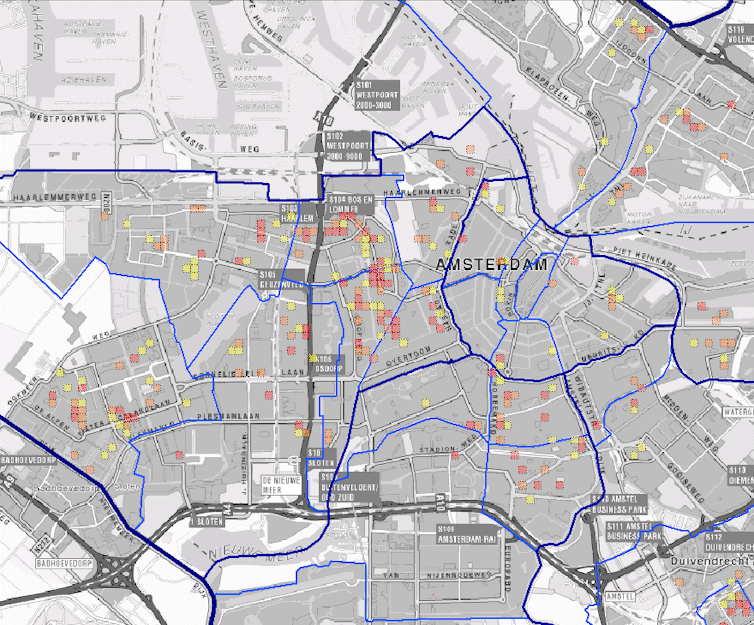
To foster inclusive practices, government practitioners could work with citizens to develop inclusive data collection protocols.
Storage
Data storage refers to where and how data is stored by the government, such as in databases or cloud data storage services. We found that government decisions about access to stored data and data ownership might lead to administrative exclusion, meaning unintentionally restricting citizen access to benefits and services. For example, administrative registration errors in applications for services and the difficulty citizens experience when they attempt to correct errors in stored data can lead to differences in how governments treat them and even a loss of public services.
We also found that personal data might be stored with cloud vendors in data warehouses outside the influence of the government organizations that initially created and collected the data. While governments are typically required to follow rigorous data collection practices, data storage companies do not necessarily need to comply with the same standards.
To overcome this problem, governments can set transparency and accountability requirements for data storage that foster inclusion.
Analysis
One important way governments analyze data to extract information is by using algorithms. For example, predictive policing uses algorithms to predict where crime will occur.
A key question is who is conducting the analysis. Those who might be providing data, such as citizens or civil society organizations, are less likely to analyze the data. Citizens may not have the skills, expertise or the tools to do so. Often, external experts conduct the analysis, and they might be unaware of the historical context, culture and local conditions of the data. In that way, data may also construct and reinforce inequalities.
To foster inclusion, governments could diversify and increase the training of the teams who perform the analyses and write the algorithms so that they can interpret data within its larger historical and political context.
Using the data
Finally, governments are using the results of data analysis to inform public service provision. For example, data-driven visualizations, such as maps, might be used to make decisions about where to direct police officers. However, this might also lead to disproportionate surveillance of different groups.
Another issue is “function creep.” Data might be collected for one purpose but is often eventually used for other purposes or by other government agencies, possibly leading to misuse of data and the reproduction of inequalities.
Digital literacy programs for both government professionals and the public can facilitate a better understanding of how data is visualized and used.
Building inclusion into the process
It is important to highlight that these activities – collection, storage, analysis and use – are linked. Inequalities in the early stages may eventually lead to inequitable outcomes in the form of policies, decisions and services.
Additionally, we found a conundrum: On the one hand, the invisibility of vulnerable groups in data collection can result in inequalities. Therefore, different groups should be included in the activities of the data process. On the other hand, this can also be problematic because digital footprints can lead to oversurveillance of the same groups.
Reconciling these conflicting concerns requires an ethical reflection: pausing before embracing data and reflecting on its purpose, limitations and long-term implications for inclusion.
The four activities are a repeated rather than linear process in which governments, citizens and third parties embrace inclusive data strategies. This means looking at what was created, including diverse voices and understanding the analysis, results and consequences of decisions. And it means consistently changing aspects of the process that do not foster inclusion.
Suzanne J. Piotrowski has received funding from the National Science Foundation and the Open Government Partnership.
Gregory Porumbescu has received external funding from the National Science Foundation and the New Jersey Office of the Secretary of Higher Education.
Erna Ruijer does not work for, consult, own shares in or receive funding from any company or organization that would benefit from this article, and has disclosed no relevant affiliations beyond their academic appointment.
Read These Next
Tiny, compact galaxies are masters of disguise in the distant universe − searching for the secrets b
These small galaxies are either crammed with stars or they host gigantic black holes. The data astronomers…
Trump campaign violated rules in Arlington National Cemetery visit, cemetery legal expert explains
Cemeteries can set their own rules, and Arlington’s are the most restrictive in the nation, meant…
Black church leaders brought religion to politics in the ‘60s – but it was dramatically different fr
In 1966, the assertion of Black power was grounded in an appeal to the founding principles of the United…